This article is the first of five (5) articles that fall under the umbrella of the paper “ISPE│GAMP5 – a risk-based approach to compliant gxp computerized systems, second Edition: PRESENTATION OF THE Major Changes” released in October 2022.
INTRODUCTION
The broad scope of digital health includes categories such as health information technology or wearable devices. Digital health technologies use computing platforms, connectivity, software, and sensors for health care and related uses. Digital tools offer real opportunities to improve medical outcomes and enhance efficiency, and give providers a more holistic view of patient health through access to data.
Within the field of digital health, Artificial Intelligence and Machine Learning (AI/ML) tools and technologies have the potential to transform health care by deriving new and important insights from the vast amount of data generated. However, AI/ML “presents the life sciences industry with a challenge in maintaining the overall quality and regulatory compliance of IT systems, applications, and/or solutions”.
GAMP5 Edition 2 introduced the new Appendix D11 – Artificial Intelligence and Machine Learning (AI/ML) (1), which provides guidance on how to ensure compliant integration and fitness for use in a validated environment.
Note: In its release of ‘ISPE GAMP RDI Good Practice Guide: Data Integrity by Design, First Edition (2), ISPE GAMP introduced the special interest ‘Appendix S1 – Artificial Intelligence: Machine Leaning’ that examines the area of Machine Learning, but which only focuses on the data integrity in AI/ML.
DISCUSSION
Artificial Intelligence and Machine Learning terms are often used interchangeably, and sometimes lead to some confusion. To put it simply, AI and ML can be defined as:
- Artificial Intelligence is the broader concept of machines being able to carry out tasks in a way that we would consider “smart”, and
- Machine Learning is a current application of AI based around the idea that we should just be able to give machines access to data and let them learn for themselves.
GAMP5 calls ML a subdiscipline of AI, and a sub-system of an application and/or business solution. It defines ML as a “method of data analysis that builds and automates mathematical models (i.e., algorithms) based on data in order to make predictions or decisions”.
The new AI/ML Appendix D11 primarily presents an overview of a risk-based compliant AI/ML life cycle framework in alignment with GAMP5 principles and phases of system life cycle. Additionally, and because an ML is fed with and uses production data or clinical data (as examples) throughout its lifecycle, carefully evaluating the risks to the quality and integrity of the data and the risks to the ML algorithm itself, is critical.
The GAMP5 diagram below illustrates the GAMP5 life cycle phases (i.e., Concept, Project, and Operation) with ML sub-system:
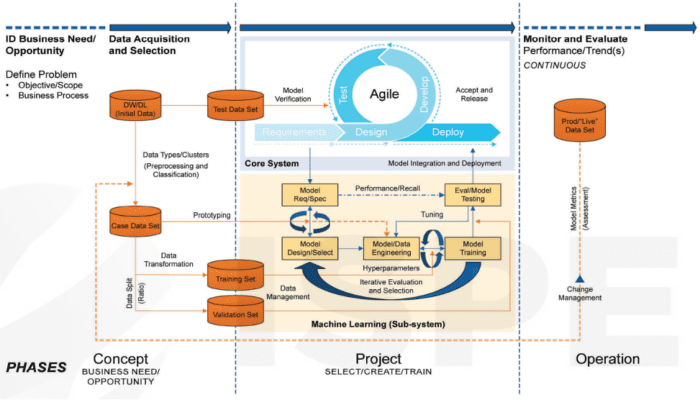
GAMP5 Appendix D11 and Appendix S11 of the Integration by Design good practice guide detail the concurrent efforts and activities to maintain data integrity and to maintain the ML sub-system itself for the duration of the system their lifecycle.
The below table is a highly simplified view of what occurs at the applicable GAMP5 system life cycle phases:
GAMP5 Life Cycle Phase |
Data Life Cycle |
ML |
|
Efforts and Activities |
|
Concept |
Data creation, identification, selection, preparation |
Selection of suitable algorithms and hyperparameters, and preliminary parameter values |
Project |
Data management activities: acquisition of new data, partition of data to be used for training, testing or validation) |
· Selected technology/algorithm and the technical architecture is specified · Iterative activities: model design, model training, testing, hyperparameter tuning · Validation UAT testing |
Operation |
· Introduce new data to the ML, as needed · Data risk assessment: impact to the data when change to the ML is applied. |
· Monitoring of performance · Risk assessment on ML in scope of change and configuration management: further configuration/coding, testing, training, and evaluation |
CONCLUSION
The use of AI/ML technologies and tools evolves rapidly and significantly in today’s Digital Health fields. With AI/ML implemented in medical imaging, in clinical decision support software, or incorporated in medical devices, AI/ML has become a focused area for regulatory bodies, as new regulatory questions are raised, and their impact across the lifecycle of regulated health products is scrutinized.
While FDA continues to release regulatory frameworks and guiding principles on the subject of AI/ML(3) , the GAMP5 Appendix D11 and Appendix S11 of the Integration by Design good practice guide provide additional and valuable information to understand how to incorporate AI/ML lifecycle management when used in regulated systems and applications.
References:
(1) ISPE│GAMP5, Second Edition, 2022, Appendix D11 – Artificial Intelligence and Machine Learning (AI/ML)
(2) ISPE GAMP RDI Good Practice Guide: Data Integrity by Design, First Edition, October 2020, Appendix S1 – Artificial Intelligence: Machine Leaning.
(3) FDA: Artificial Intelligence/Machine Learning (AI/ML)-Based Software as a Medical Device (SaMD) Action Plan, January 2021.
(3) FDA: Good Machine Learning Practice for Medical Device Development: Guiding Principles, October 2021.